Improving the Segmentation and Visualization of Intracranial Aneurysms in 3D Angiograms
When segmenting and visualizing intracranial aneurysms in three-dimensional (3D) angiograms, there is often a tendency to overemphasize the neck of the aneurysm, whereas its nature plays a crucial role in the morphological analysis for selecting the appropriate interventional procedure. Using two-dimensional (2D) angiographic imaging, the true morphology can be more accurately assessed if the projection direction is appropriate. On the one hand, such 2D data are obtained in the form of additional high-resolution images during diagnosis or intervention. On the other hand, they are available in the form of lower resolution 2D raw data from the acquisition of the 3D images themselves.
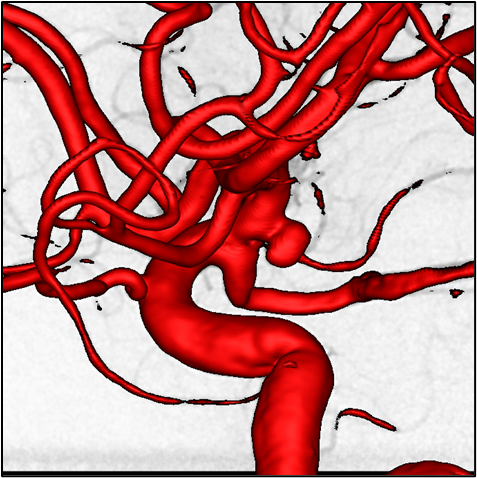
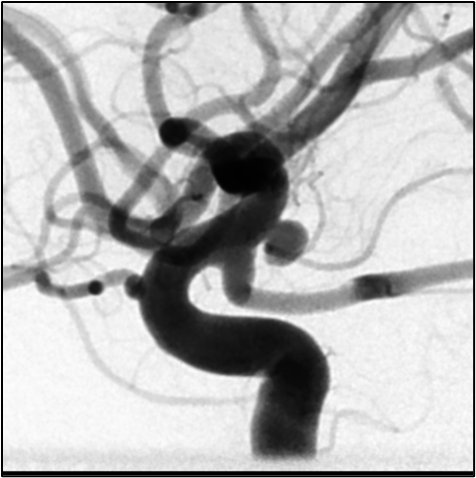
In this thesis, both types of 2D images will be tested for their suitability to be used as an automated mask for the optimization of 3D visualization and segmentation in order to improve the morphological quality of 3D visualization and segmentation.
The implementation will be done in Python using the open source tools Visualization Toolkit (VTK – https://vtk.org) for segmentation and visualization and if necessary Insight Toolkit (ITK – https://itk.org) or SimpleITK (https://simpleitk.org) for any necessary registration between 2D and 3D image series.
Type: Bachelor/ or Master Thesis
Requirements: Good skills in scientific reading; critical thinking; good skills in programming (Python, VTK and related toolkits)